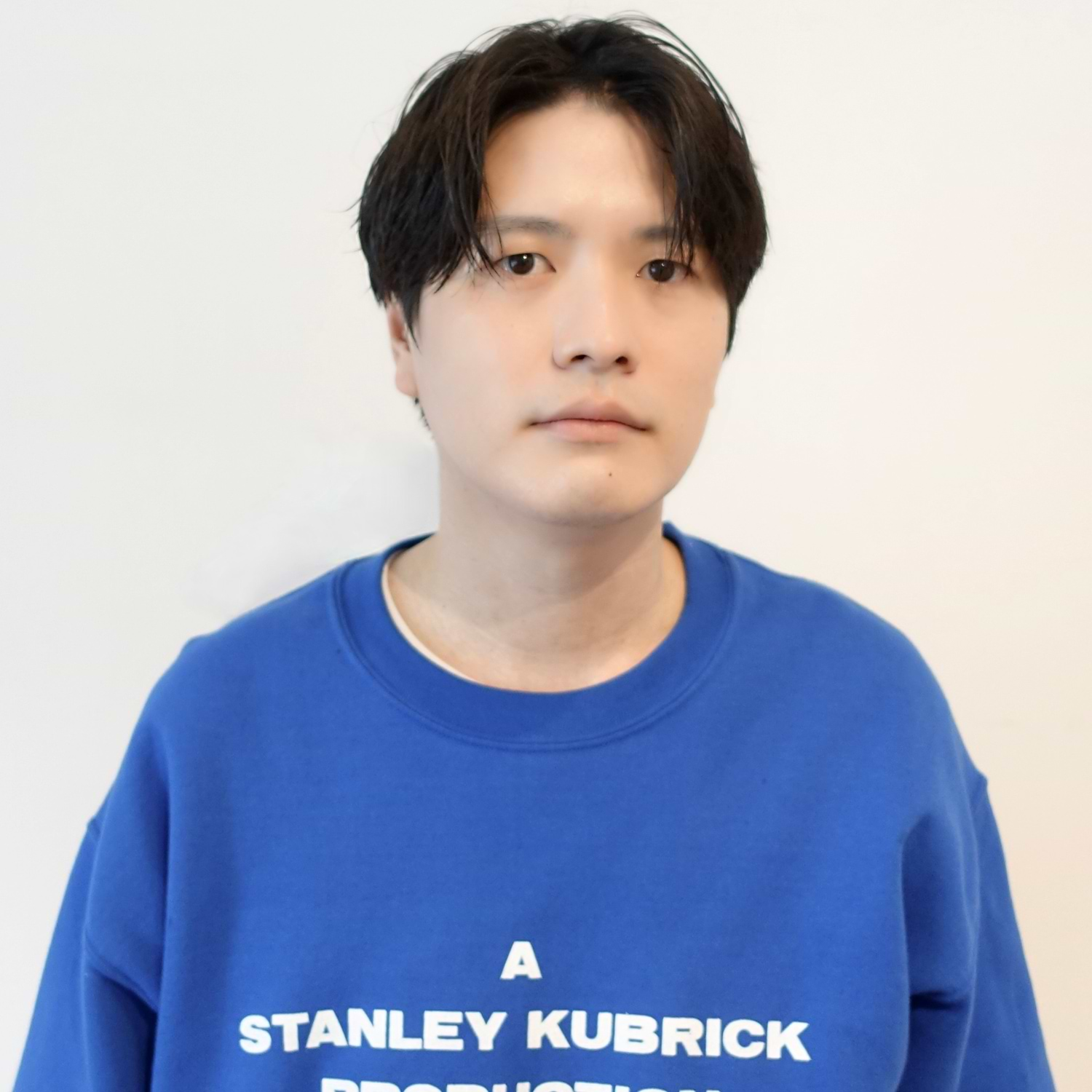
Dr. Konosuke Noto
Data Scientist
Data generated by digitalized products, services, and operational procedures are being used to reform businesses and improve processes that are regarded as important components of digital transformation.* In this article, I discuss how this is relevant to marketing, as well as Dentsu’s related capabilities and initiatives.
* | Japan’s Ministry of Economy, Trade and Industry defines digital transformation as the application of data and digital technologies by companies for the improvement of their products, services, and business models. Improvements are based on consumer and societal needs, and companies’ requirements to enhance their operations, organizations, processes, and corporate culture in order to secure a competitive edge. |
Digital transformation projects see little success
While all kinds of things are being digitalized today, users often say digitalized products and services are difficult to understand and use. Thus, countless databases and business intelligence tools are largely unused. In fact, the success rate of digital transformation projects in Japan is only around 15% (and even lower for projects carried out at long-established companies), according to a consulting company’s report (see reference materials 1 and 2).
Although people tend to imagine a bright future when they think of digital transformation, we need to remind ourselves of how extremely difficult it is for businesses to successfully execute new projects. With this in mind, I would like to discuss how digitalization can be beneficial.
Role of data in trial-and-error process
Digitalization by itself does not guarantee success. Nevertheless, by digitalizing a product, for example, we can obtain and accumulate data on how the product is really used by specific users. On that basis, we can take steps to make improvements and to innovate. We can also use the data to monitor the results of those actions, and create a feedback loop incorporating both the data generated and the decisions made.
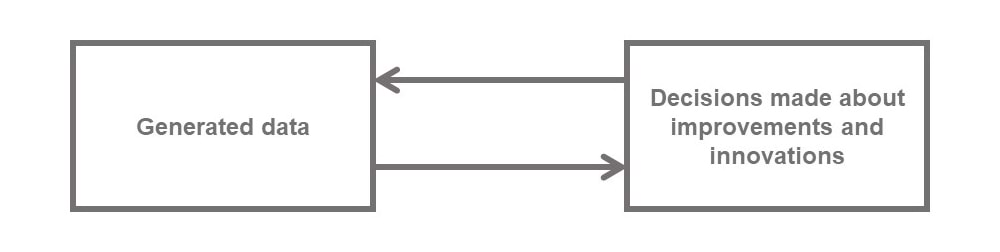
I believe the trial and error process enabled by the feedback loop is a major benefit of digitalization. Traditionally, decision-making has relied on intuition and experience. In contrast, data-driven decision-making involves trying out an improvement, and then either abandoning it or making another one based on the data results.
In other words, the data provides an objective standard and a basis for making decisions. This allows people not only to make better decisions, but to make them more quickly. As a result, when decisions are made based on data, they do not have to end in failure. In my view, this is an important advantage of digitalization. Yet, to facilitate decision-making, several factors are critical.
Aligning variables using data-driven decision-making
Data-driven decision-making can be broken down into specific stages, as shown in the following diagram.

Analysts and data scientists pay attention to analytical methods when using data, such as statistical analysis and machine learning estimates. However, a variety of other factors also need to be considered by project teams wanting to effectively use data when making business-related decisions.
For example, data generated over the course of digitalization are not produced for analytical purposes. They are derived from logs and other sources that can be acquired through routine business processes and, therefore, contain various biases. Some of these may not be accurately identified by an analysist.
Further, since the quality of data can have a major effect on a project as a whole, the team should create an analytical design—that includes data preprocessing and the planning of analytical methods—while considering the degree to which the data reflects reality.
To accurately address questions raised by the analysis, basic knowledge of data science is required in order to comprehend the assumptions and conditions underlying various analytical methods.
This means the results of data analysis do not reflect the object of the analysis but, rather, a combination of the object, the data generation process, and the analytical design.
Accordingly, if only the results of the analysis are used without an understanding of how the results were generated and how the analysis was designed, there is a risk that bad decisions shall be made.
It should also be noted, however, that even if proper data analysis procedures are followed to confirm the validity of the data, whether the results actually contribute to effective decision-making about business matters is another issue.
Analytical questions must be specified based on business-related issues and knowledge of the implementation tools, while business issues can be identified by examining data.
But the understanding of implementation tools is limited by many business-, legal-, and system-related constraints. So, if the impediments are not addressed by the analytical questions, reflecting the results in implementation will be difficult.
For this reason, it is important to link the analytical design and implementation design components of the analytical questions.
Indeed, to finally devise solutions for business issues through data-driven decision-making, various factors must be integrated. (Similarly, AI is designed as a single system comprising multiple factors.) Because data cannot provide a single path to a solution, it needs to be aligned. This makes solving business issues hard work, requiring that data scientists and analysts in project teams repeatedly examine each factor while constantly trying to align everything into a series.
When creating a series, the project team should have many options for making adjustments to the analytical methods and implementation tools. But in practice, the scope for adjusting the methods for analyzing given data is not wide, so it is important that there be numerous options for implementation.
Fortunately, Dentsu has not only extensive experience in executing marketing and communications campaigns, but also established systems for developing many kinds of implementation tools and businesses, as well as organizations, products, and services. These wide-ranging capabilities are very effective for the work of alignment I mentioned earlier.
Understanding and predicting key causal relationships in decision-making
So far, I have explained the reason data-driven decision-making is difficult and how, when done properly, it can increase the effectiveness of steps to make improvements and advances through trial and error.
In this regard, the difference between correlation and causality is a key point. It is well understood that two phenomena can be correlated in a data set, but that it does not necessarily mean the two are causally related. So when making decisions about business, causal relationships are very important.
A good example is the relationship between ad placements and sales results. When checking data showing either an increase or decrease in sales during the time an ad is placed, people often get confused about whether these results are correlated or causally related to the effectiveness of the ad campaign.
In some cases, sales are not a result of advertising, but of other factors (called confounding variables) present at the time the ad was placed. Conversely, sales might not increase due to other factors, even though the advertising was effective.
Even today, one finds decisions being made regarding future marketing budgets based on conclusions about the effectiveness of prior ad campaigns, without any understanding of causal relationships.
In this regard, causal inference is effective for improving routine decision-making. Causal inference uses statistical analytical methods for measuring the direction and magnitude of cause and effect, as well as of experimental methods such as randomized control trials.
Quasi-experimental methods are used when experiments are difficult to conduct. Well-known methods include propensity score matching, difference in differences (fixed effects model), counterfactual simulation, instrumental variable, and regression discontinuity design.
None of these methods can be used unconditionally but, if the best method for the usable data and analytical questions is chosen, it is possible to reach well-grounded conclusions about whether certain actions were effective and, if so, to what extent. It is also possible to make predictions about the extent to which similar actions could be effective.
As an example of how causal inference is applied, below I briefly describe how we analyzed purchasing behavior using customer IDs and point-of-sale data obtained from a major retailer’s official app.
The client was using the customer relationship management app, the users of which numbered among the highest in Japan. The client understood the impact of each component of an advertising campaign, as measured by their respective key performance indicators (KPIs), by using randomized control trials, propensity score matching, and other methods.
Nevertheless, the client was not able to confirm whether the components of the campaign had contributed to an increase in the overall amount purchased, which was the key goal indicator (KGI). Since the impact pathways from the KPIs to the KGI were unclear, the cost effectiveness of the ad campaign could not be calculated because the effect on the final purchase amount was uncertain.
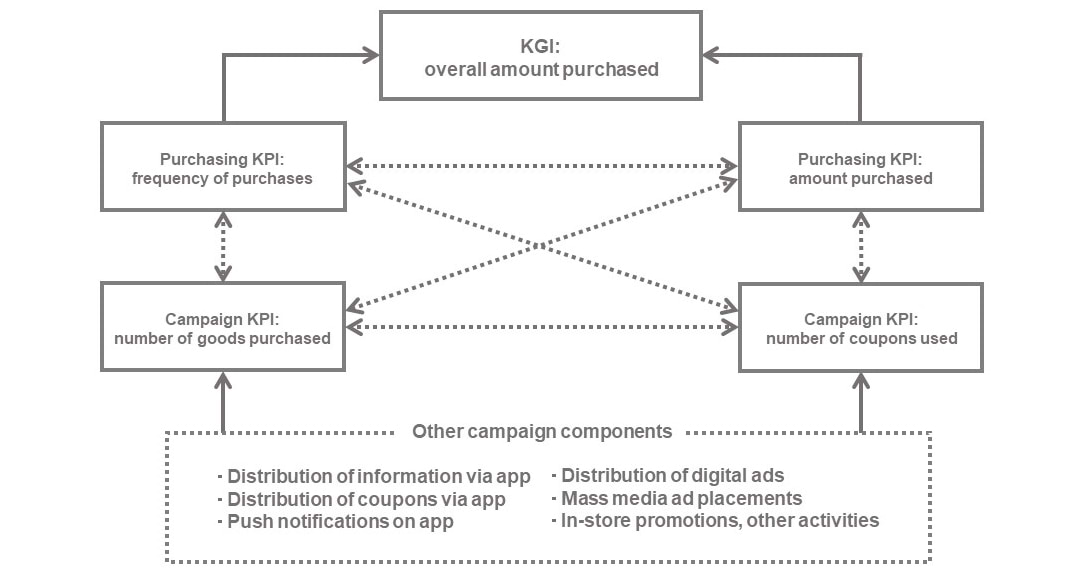
To solve this issue, Dentsu’s project team examined the connections between each component of the campaign and the KGI (see diagram 3), and measured the causal relationships between the KPIs, in terms of both direction and magnitude (as indicated by the dotted lines).
Panel data (behavior of individual users across time) for the app’s users were created to control several factors, specifically the high correlation between the results for the KPIs and their mutual impact on each other (called endogeneity), as well as the variability of in-store shopping behavior, which is greatly influenced by season, store location, and personal shopping preferences.
The team then conducted an analysis using a combination of several causal inference methods. The analysis showed that certain components of the ad campaign contributed to increasing the overall amount purchased (the KGI), while other components did not; in fact, they had a negative effect. Other combinations of components only had an effect among certain cohorts of the app’s users. Since we obtained these findings by applying a sound controlling method for external variables, we were able to estimate the cost effectiveness of each component of the advertising campaign. Furthermore, this analysis provides a model for predicting the effect of each action on a specified KGI, allowing us to understand the effectiveness of the actions in advance.
To offer this kind of expertise in causal inference and problem solving as a standard service in all data projects, the Dentsu Group is putting together project teams led by data scientists and analysts (see reference materials 1 and 2). By having data scientists and analysts participate from the initial meetings and project execution stage, in particular, their data analysis expertise can be reflected in a project’s implementation. Further, they also can propose to incorporate a plan-do-check-act cycle in the project as a whole, in order to obtain the data needed for the next round of decision-making.
Hard facts, ideal scenarios
So far I have discussed several data applications that are important and useful for digitalization. But data-driven decision-making also involves risks and uncertainty, simply because data from the past does not necessarily mirror the future. Bearing in mind that intuition and experience long have provided a basis for making business decisions, there is no guarantee that data-driven decision-making will perform better over the short term.
Nonetheless, the data-driven decision-making process can clarify the steps being taken and provide a project team with a solid basis for answering questions about what to do with the results and how best to proceed.
Therefore, over the long term, I believe it can contribute to business growth, as long as the team continues to verify that the trial-and-error process is moving in the right direction while the project is being carried out.
Accordingly, it is important to foster an organizational culture that understands and appreciates the work processes involved in digitalization and data projects. It is also vital that progress be evaluated from a long-term perspective.
The Dentsu Group has declared its commitment to helping clients achieve growth over the long term by combining its many solutions as an integrated growth partner. I believe data will play a central role in these endeavors as we continue developing data-related solutions.
To improve its data analysis capabilities, the Dentsu Group has established Dentsu Cross Brain Inc. in cooperation with BrainPad Inc. And, ahead of other companies in Japan, in order to continue building up expertise, the Group is utilizing data clean rooms operated by major platforms, such as Google, Facebook, and Amazon.
Further, to offer solutions to clients selling everyday consumer goods, around 90% of which are still sold in stores in Japan, we are supporting the development and operations of retail media marketing platforms that integrate major retailers’ purchasing data and a variety of implementation conditions. The data solutions are somewhat limited at present but, as we continue to refine and master them, I believe our clients will come to recognize the benefits of data-driven decision-making.
Finally, achieving a digital transformation is a challenge for businesses rather than for technology. Decision-making that starts from data can, I believe, serve as an effective way for businesses to become more competitive. Nonetheless, it should not be treated as doctrine. This is especially true when data-driven decision-making starts to take root in a team, as its members could become fixated on numerical results and ignore information that cannot be accurately captured by data.
Were this to occur, it would make flexible decision-making more difficult. In contrast, intuition- and experience-based decision-making allows members to freely discuss ideal scenarios based on weak evidence and intuitive hypotheses, and this can actually be advantageous. Thus, we should first apply intuition when creating conditions for testing hypotheses with data, and explore ways to combine the hard facts derived from data with the ideal scenarios based on intuition and experience. I believe that will lead to better organizational decision-making.
Author
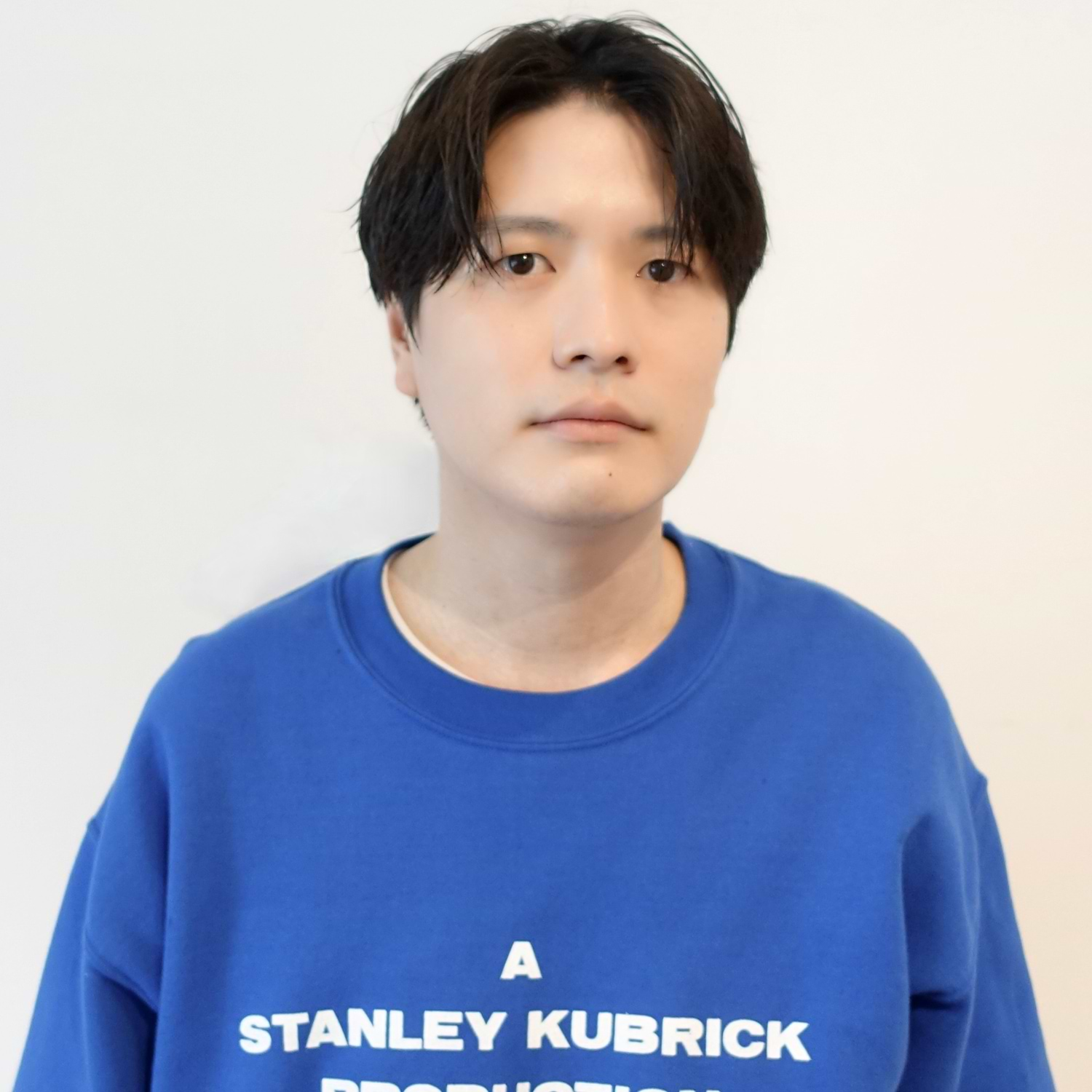
Dr. Konosuke Noto
Data Scientist
Dr. Konosuke Noto joined Dentsu in 2017 and was assigned to the Data Technology Center. He has been applying Bayesian statistics in marketing mix modelling and developing solutions for Dentsu’s people-driven data management platform. He also oversees the development and operations of Dentsu’s integrated retail media marketing business that utilizes data supplied by clients, specifically customer relationship management and purchasing data from major retailers. He holds a PhD in Policy and Media.