
Sohei Mitani
Data Technology Center
General Manager
When it comes to media, such as television and newspapers, advertisers are limited to purchasing advertising slots. But, a full-page ad in a newspaper looks the same no matter who sees it.
But in the world of digital advertising, all this changes. Auctions are held each time an ad is displayed, and the ad shown is that submitted by the auction winner—the company. This ensures that all digital media users see the ads most appropriate for them. In other words, users see different ads even when looking at the same advertising spaces.
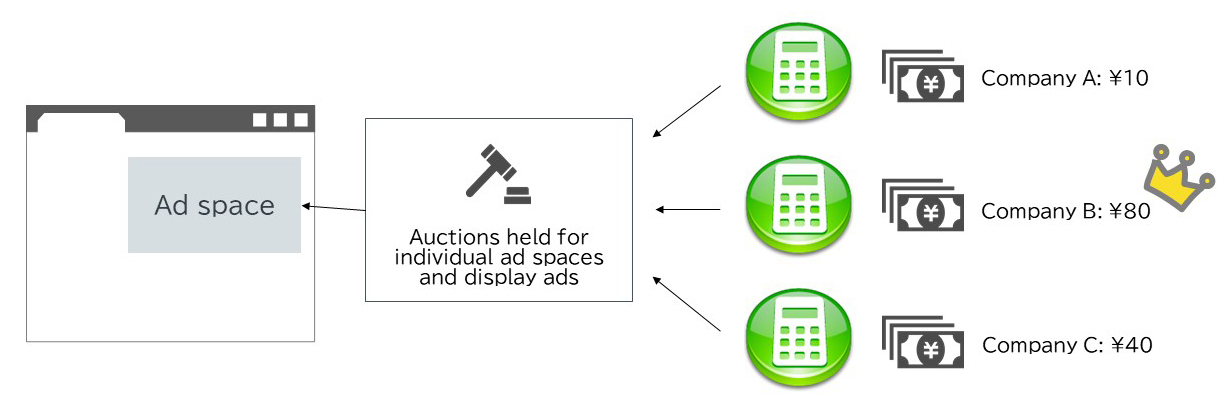
Since an auction occurs before each ad is displayed, millions or even tens of millions of bids must be handled 24 hours a day, 365 days a year. Since humans cannot handle such vast quantities of bidding, bidders use automated bidding systems that apply machine learning to predict which users are likely to require which ads. This is based on previously collected data and determines optimal bid amounts based on the probability of a request being submitted.
Put another way, the algorithm of an automated bidding system determines the optimal bidding amount by calculating, in real time, the probability of a user submitting a request. This, in turn, is based on a given user’s attributes. They include the device being used, the user’s location, the day of the week, and time of day.
In the past, operators determined their bidding amounts based on a set of rules. But, with the advent and evolution of these algorithms, operators not using automated bidding systems have become unable to compete in terms of advertising efficiency. Accordingly, ever greater numbers of operators are automating their bidding systems.
The question now is: Does the potential exist for operators to add value in this environment?
Aware of the question, Dentsu and Dentsu Digital have explored innovative ways to add value, even given the current prevalence of automated bidding.
The automatic mechanisms that determine bidding amounts are created independently by individual platform operators and, therefore, do not allow for direct intervention by external parties.
However, training data associated with models created by platform operators (i.e., whether request submissions are present or absent) and other relevant results can be forwarded externally.
So, we arrived at the idea of manipulating this externally forwarded training data to further improve advertisers’ business results amid the ongoing application of automatic bidding systems used by platform operators.
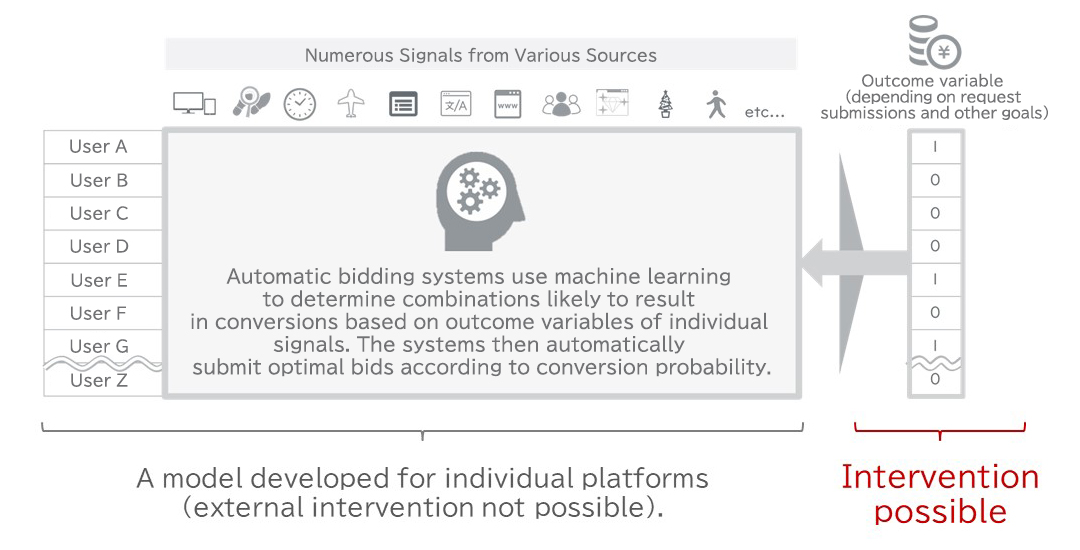
Automated Bidding Algorithms Fed Training Data Gaps
Bearing in mind that the training data targeted for learning by automated bidding systems can be manipulated, even though intervention is not possible in the automated bidding algorithms themselves, we shall now examine some challenges that emerge when conducting actual ad operations.
In general, digital advertising aims to inspire actions that can be completed using only a website. An e-commerce business might target member registration, a credit card company might aim for card issuance, and a real estate company might pursue reservations for visits to its premises.
However, the primary goals of the overall operations associated with these advertisers differ, and website-based actions are only a part of the customer journey. An e-commerce site would probably target ongoing purchases (lifetime value), while a credit card company might aim to encourage spending using their cards, and a real estate agency would indubitably strive to close deals.
In short, digital advertising tends to target more surface-level goals, while the primary goals of advertisers are generally deeper. This disparity exists in part because of gaps that exist between website-based actions performed by users and the subsequent business outcomes targeted by the companies operating the sites.
An e-commerce site cannot secure ongoing purchases on the same day that a customer registers; it only can do so after a certain period of time—perhaps six months. Yet, the automated bidding algorithms of individual platforms are often developed to acquire information about behavior occurring within a few days following a click. That means the algorithms will not generate adequate information if it is transmitted too late (maybe six months after the initial click).
Gaps between website-based user actions and subsequent business outcomes are not only time-based, but quantitative. Automated bidding algorithms predict the future by learning from existing data. If too little historical data is available, learning can be insufficient and the accuracy of the corresponding model can be compromised.
Thus, if a health food business initially offers a product free in a promotional campaign, and one percent of those participating in the campaign go on to purchase the product (a conversion rate of one percent), the business would only generate 100 subsequent purchases were 10,000 people to take advantage of the free trial.
But if, as in this example, there is a small sample set of business results and they directly correspond to the training data of the automatic bidding algorithm, the sample set may not generate adequate learning. This would cause the automated bidding system to function improperly.
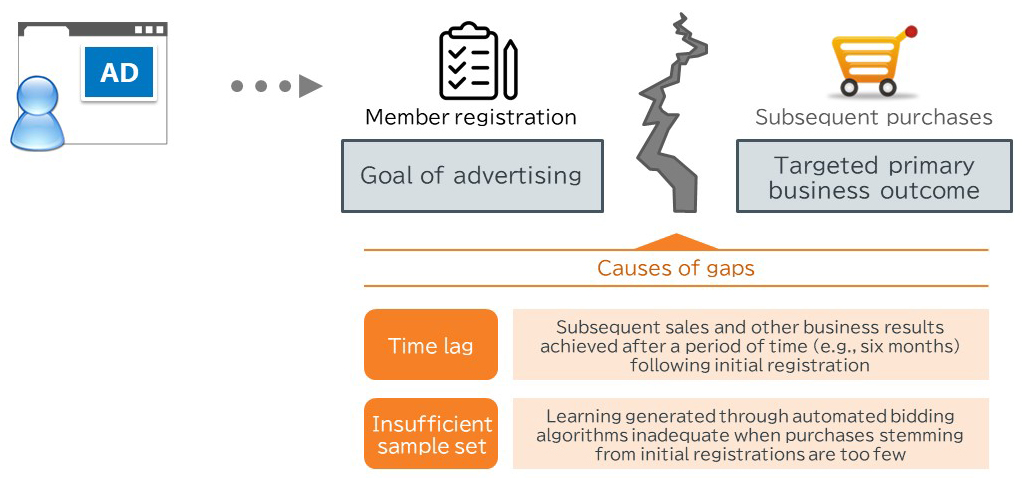
Closing Gaps
This information indicates that the primary business results targeted by businesses should be designated as training data, because they are the optimal targets for automated bidding algorithms.
However, we found that in many cases, direct optimization is rendered impossible due to time- and volume-related gaps. Companies must thus set up measurement points on their websites.
Dentsu and Dentsu Digital have responded to these challenges by aiming to close the gaps through an analytical technique called predictive modeling. This has allowed us to develop models that enable us to predict the probability of achieving primary targeted business results, such as subsequent purchases in the case of an e-commerce site (i.e., individual user value), through the application of data initially available (at the time of initial member registration), past data sets, and probable future data.
In other words, through predictive models, we can project the probability of subsequent behavior (targeted primary business results) through analysis of past website-based activity.
By submitting projected user value as training data for advertising platforms, we were able to maximize the achievement of targeted primary business results.
Time- and volume-related gaps between website-based user activity and subsequent business outcomes affect not only e-commerce sites, but also elements of other industries. The gaps also affect subscriber retention rates following initial registrations, and purchase rates following the initial activation of apps. Accordingly, predictive models provide a wide range of possible solutions.
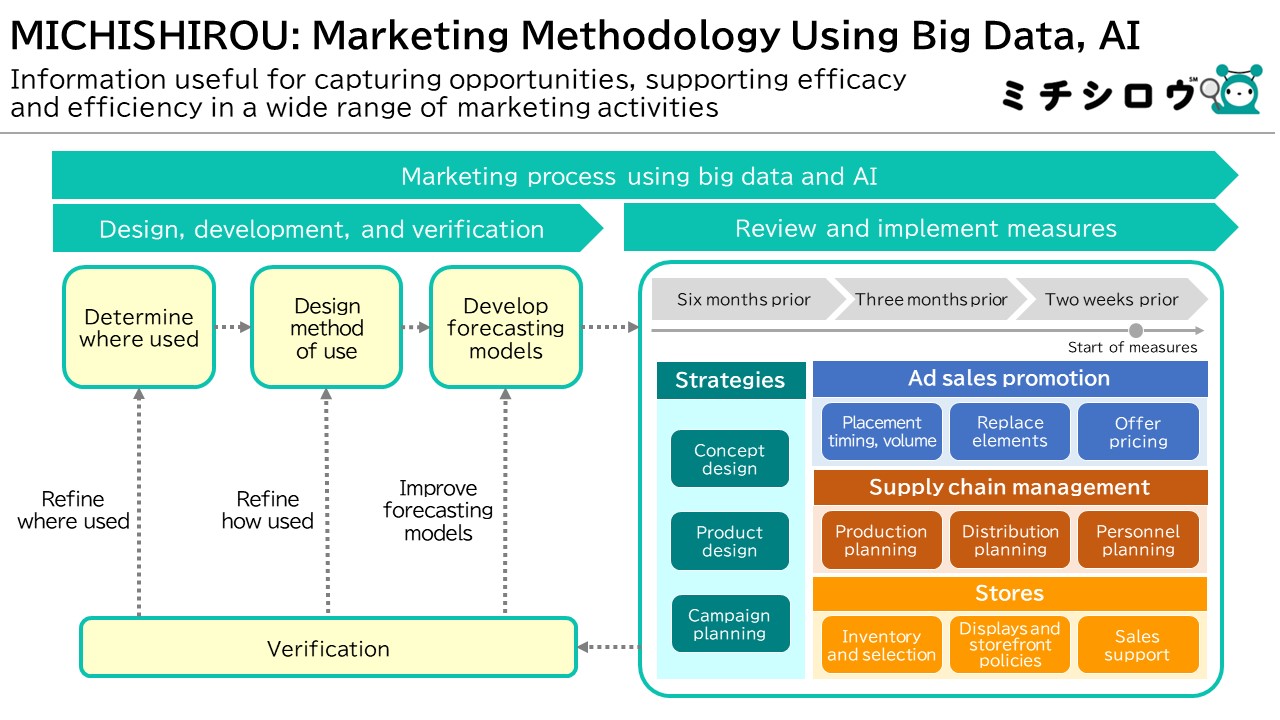
How Are Predictive Models Created?
When setting up a predictive model, we first consider what data can be used and what business outcomes can be estimated to maximize marketing effectiveness. If necessary, we also provide advice and assistance related to the acquisition of new data, while offering proposals concerning the preparation of new medium-term indicators.
Once data has been collected, it is incorporated into the cloud platform. The data used is varied but, primarily, includes web access logs, application data, and membership data (demographic information and purchase data).
Once the relevant data has been gathered, machine learning and predictive models are created. We ensure a satisfactory level of accuracy by establishing a schedule, comprising multiple test runs, and determining features to be incorporated.
Once the model has been created, we calculate projected scores (predicted lifetime value in the case of sales) for each user. These scores are linked to their respective advertising platforms through an application programming interface (API) and used as training data for automated bidding.
Finally, we develop this series of processes into a full-fledged data pipeline. Predictive scores fluctuate depending on the specific actions of individual users. For this reason, the automation of processes associated with daily score updates and the merging of current scores into their respective ad platforms is of critical importance.
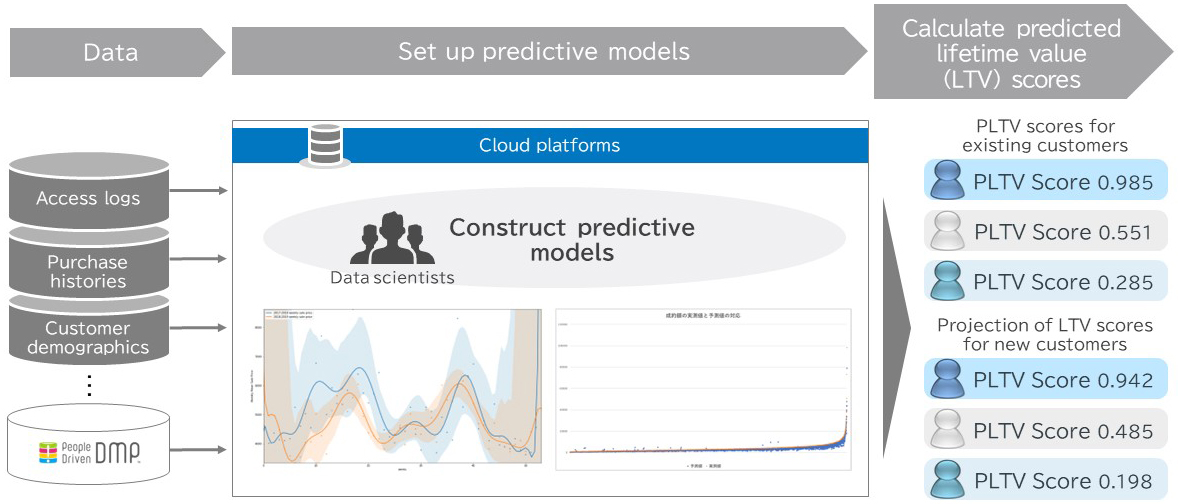
Dentsu and Dentsu Digital’s Technical Innovations
Our technical innovations are distinguished by three distinctive characteristics.
1. | Data pipeline compatible with multiple advertising platforms |
---|---|
We have developed a versatile pipeline that enables the integration of data, the assignment of predictive scores, and the incorporation of data into advertising platforms. Several existing advertising platforms can apply scores from external sources as training data for automatic bidding systems, and all of these platforms require the use of different APIs. However, platforms operated by Dentsu and Dentsu Digital can support the application of all external scores. |
2. | Seamless Coordination with Teams Running Ad Operations |
---|---|
Even if a system capable of applying scores to automated bidding systems using machine learning can be set up, it will not improve marketing effectiveness and business results without practical knowledge concerning how to conduct advertising operations. Dentsu and Dentsu Digital have accumulated this type of expertise through many past projects, enabling seamless integration of systems and operations. |
3. | R&D Efforts Focus on Data Drift, Predictive Models |
---|---|
Through collaboration involving both industrial and academic entities, we have improved the accuracy of our lifetime value projections and added time–series analysis technology, thereby making possible the creation of models capable of capturing the seasonal tendencies associated with individual consumers. Concerns are often raised regarding the degradation of machine learning models caused by changes in the distribution of data that have occurred as a result of the COVID-19 pandemic. Dentsu and Dentsu Digital have responded to these concerns by incorporating mechanisms for detecting changes in data distribution (data drift), thereby making systems more robust. |
Dentsu and Dentsu Digital aim to continue providing a variety of services and solutions that facilitate the generation of essential improvements in business results through marketing investment.
Author

Sohei Mitani
Data Technology Center
General Manager
Sohei Mitani has managed direct advertising sponsors and assisted with the digitalization of marketing processes. He is currently developing tools and promoting advances in digital marketing based on quantitative performance results.